What does fairness mean for AI systems?
In the last decade, large-scale AI systems have been deployed on an unprecedented scale to make decisions for, or about, people with little human oversight. A lot of the most successful automated decision-making systems today are based on probabilistic models trained on large amounts of data. Models are incentivised to mimic a dataset of past examples and have the capacity to accurately reproduce or even amplify existing societal biases present in the data.
Even a rigorously tested model can reveal unintentional, unfair blind spots when deployed in the real world. Biases in data seep into model parameters and model predictions, which in turn influence the model’s data generating process, i.e. the real world. Properly trained large-scale AI systems often merely reflect the biased, flawed, and opaque decision-making of humans. While mildly annoying for Netflix recommendations, outcomes can be disastrous for use cases like politically motivated social media nudging and critical applications like health care and justice. It is our responsibility to act to prevent this from happening.
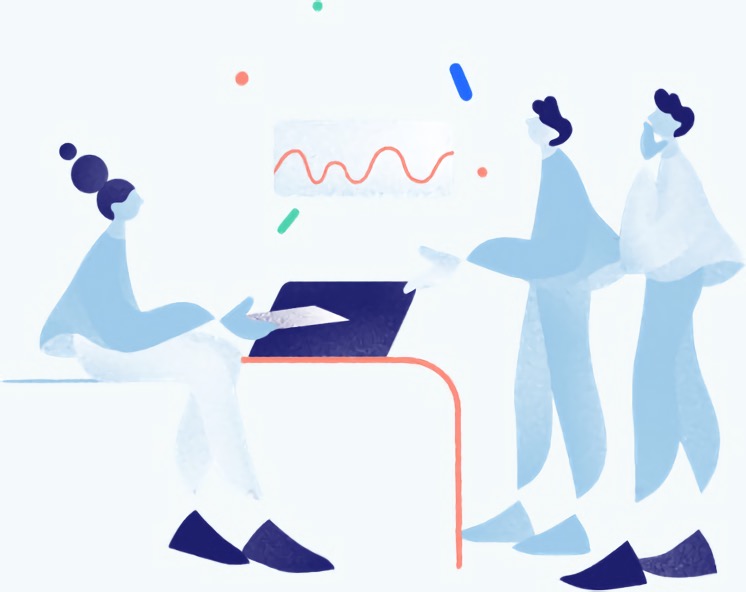
Fairness in AI is all about what we should do. There is no standard definition of fairness, whether for human or machine decisions. In the context of machine learning, fairness is actually a placeholder term for a variety of normative egalitarian considerations. Designing fair algorithms is designating some actions or outcomes as good or desirable or permissible and others as bad or undesirable or impermissible. The real challenge of fairness in AI hence lies not so much in pursuing technically satisfying fairness frameworks but rather in designing algorithmic systems compatible with human values.